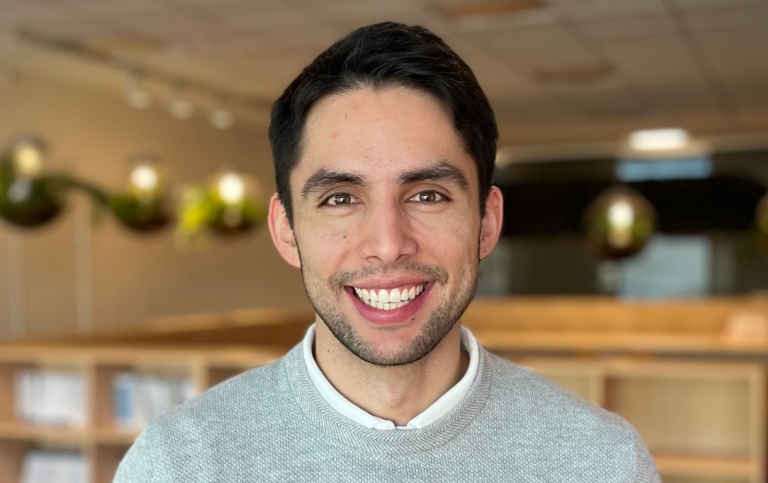
A new wave of wearable devices will collect a mountain of information on us. And there are privacy implications, writes Luis Quintero in The Conversation.
A new wave of wearable devices will collect a mountain of information on us. And there are privacy implications, writes Luis Quintero in The Conversation.
Almost 50 people came to Panagiotis Papapetrou's Tech Tuesday seminar on AI in healthcare.
Data is available in abundance. However, to utilize this raw material we need to sort it so that patterns become visible.
Meet Simon Hacks, new associate professor at DSV. His next project involves optimising maritime transports.
For most of us, "the cloud" is kind of fuzzy. We don’t know where it is – or how safe it is. Ramin Firouzi identifies two major problems with cloud computing.
“A self-driving car constructs a simplified picture of the world,” writes Barry Brown in The Conversation.